By leveraging AWS IoT and cloud services,RayRay Manufacturing successfully transformed their manufacturing operations. Lets show you how?
Who is RayRay Manufacturing?
is a leading manufacturer of industrial paintbooth. They operate multiple manufacturing facilities across the United States and have a commitment to innovation and sustainability. RayRay Manufacturing faced challenges in optimizing their manufacturing processes, reducing downtime, and enhancing product quality.
is a leading manufacturer of industrial paintbooth. They operate multiple manufacturing facilities across the United States and have a commitment to innovation and sustainability. RayRay Manufacturing faced challenges in optimizing their manufacturing processes, reducing downtime, and enhancing product quality.

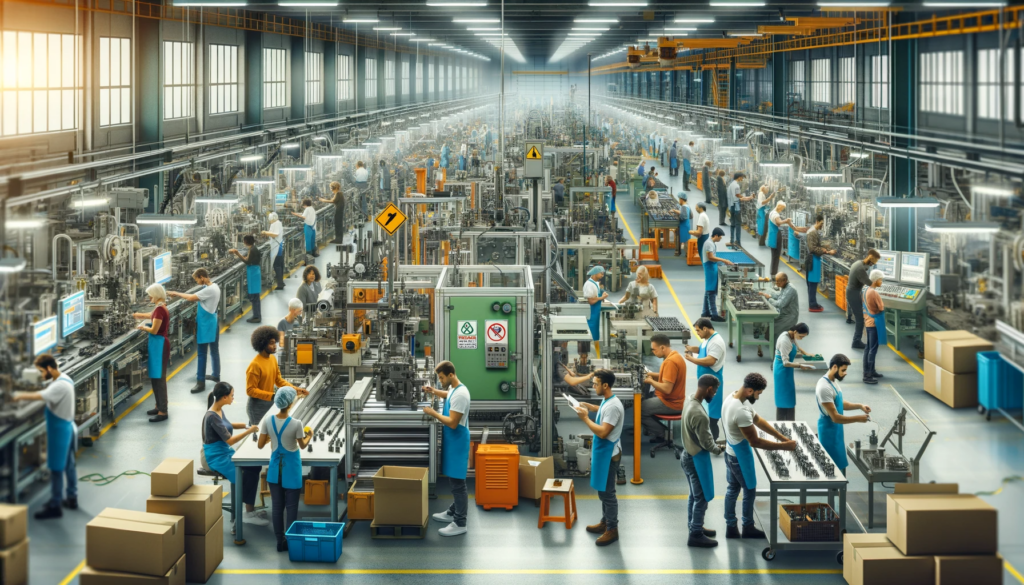
The Challenge
One of the significant challenges that RayRay Manufacturing faced was the pervasive issue of unplanned downtime and maintenance disruptions within their manufacturing operations. Unplanned downtime, often resulting from equipment failures or unexpected maintenance needs, had become a persistent obstacle to their production efficiency. This downtime not only caused delays in fulfilling customer orders and increased operational costs but also substantially diminished the overall equipment effectiveness (OEE) of their manufacturing facilities. Frequent, unexpected shutdowns not only hindered the company’s ability to meet customer demands but also strained resources, as the time and manpower required for unscheduled repairs and maintenance were substantial. Furthermore, these interruptions posed a significant challenge to workforce productivity, making it challenging to achieve optimal utilization of equipment and resources. In essence, the pervasive issue of unplanned downtime was not only a financial burden but also a hindrance to Ray Ray Manufacturing competitiveness and its ability to maintain a streamlined and cost-effective manufacturing operation.
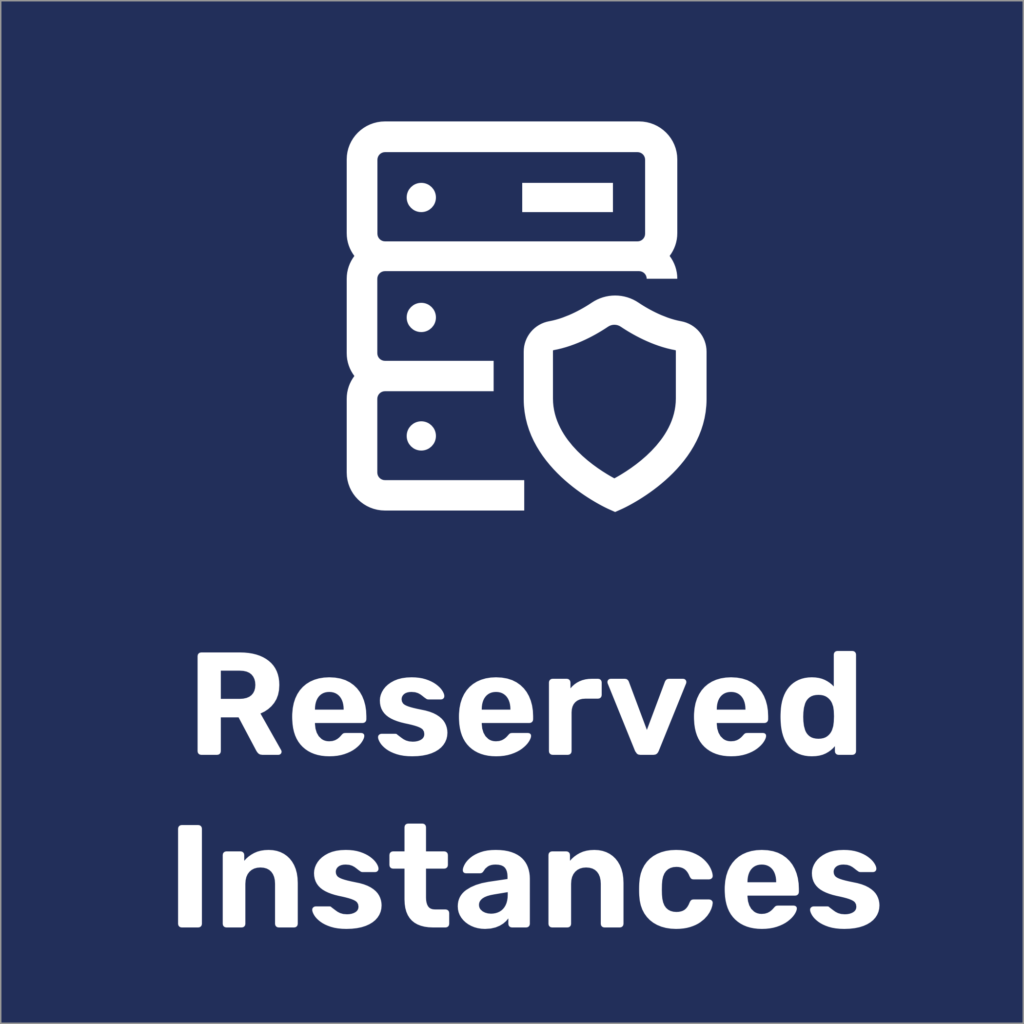
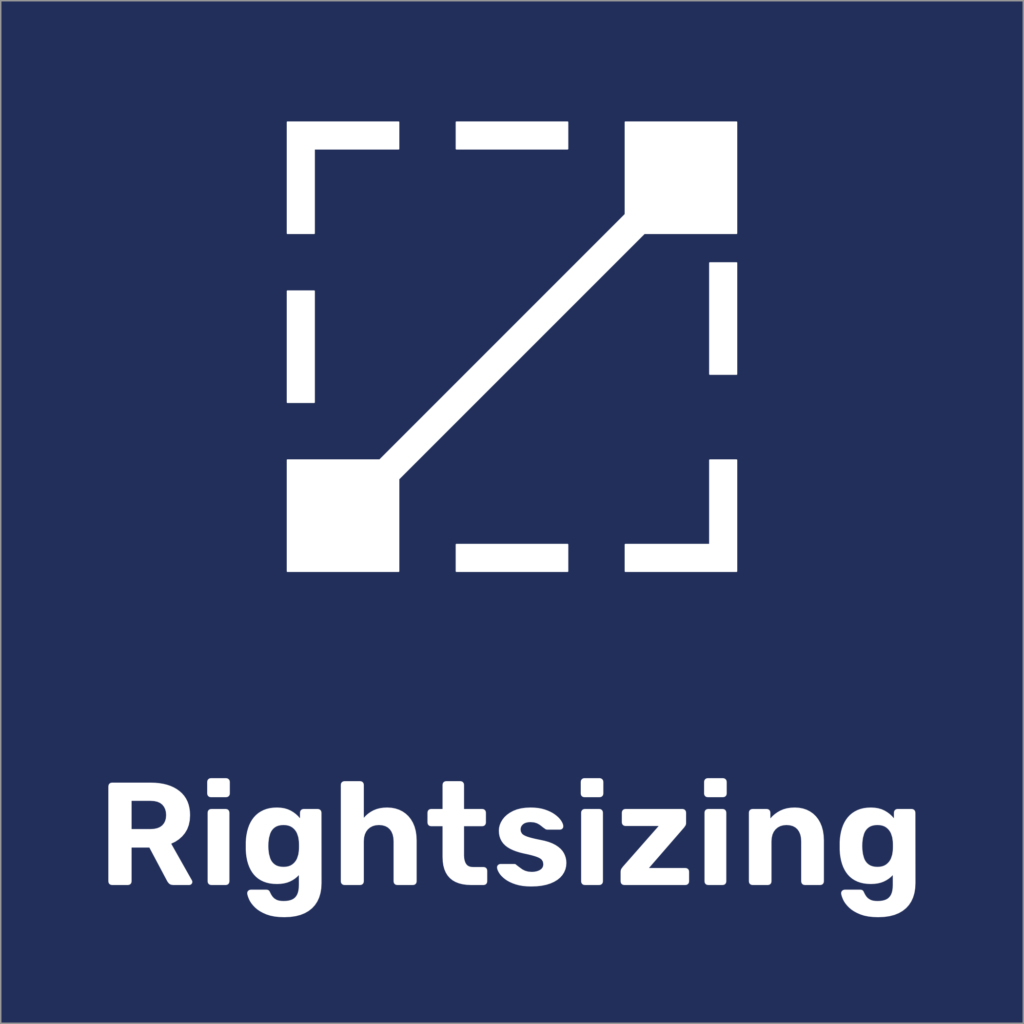
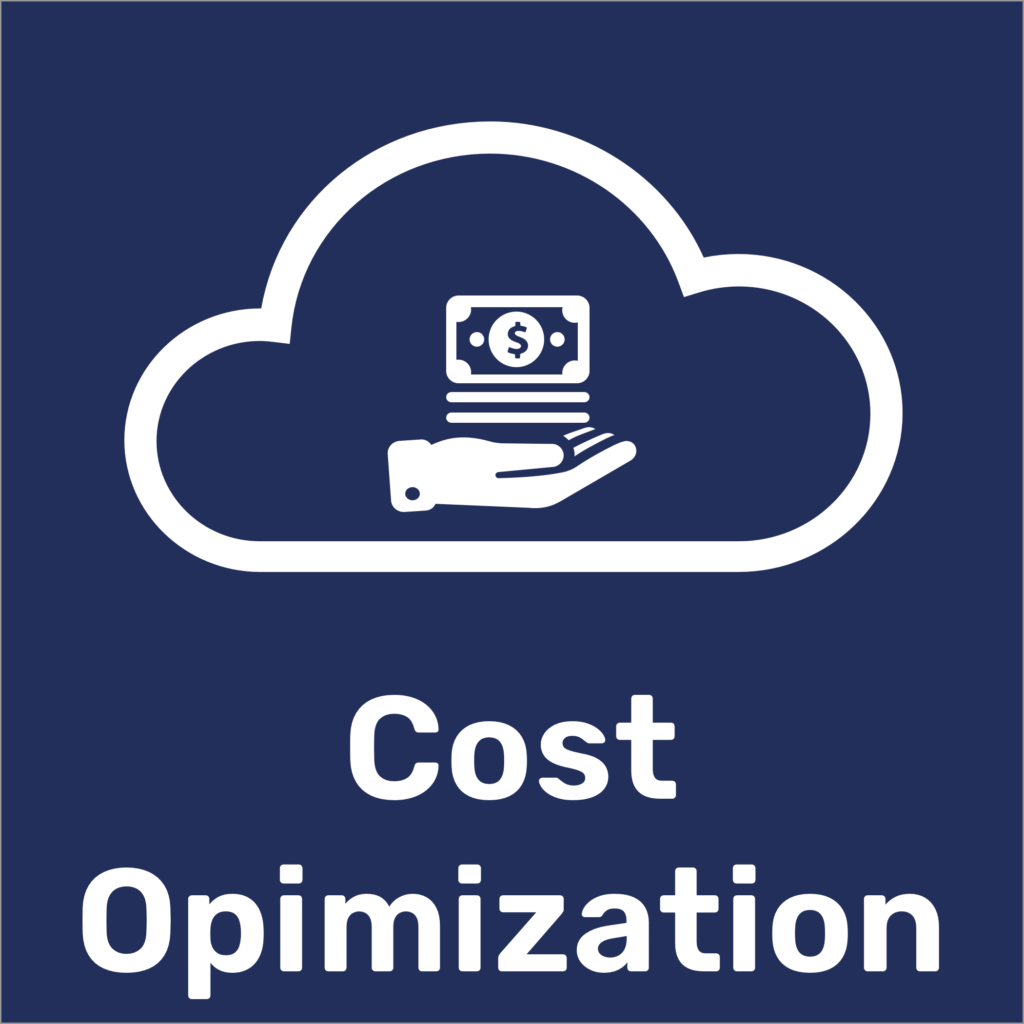
The Solution
IoT Device Integration: ShireCloud deployed IoT sensors and devices on manufacturing equipment to collect real-time data on machine performance, temperature, energy usage, and more.
AWS IoT Core: ShireCloud used AWS IoT Core to securely connect and manage their IoT devices. This allowed them to ingest large volumes of data from the factory floor into the cloud.
AWS Greengrass: AWS Greengrass was deployed at the edge, enabling localized processing of data, reducing latency, and allowing for real-time decision-making on the factory floor.
Amazon SageMaker: ShireCloud employed Amazon SageMaker to develop machine learning models for predictive maintenance. These models analyzed data from IoT devices to predict equipment failures and maintenance needs before they occurred, reducing downtime and repair costs.
AWS Lambda: AWS Lambda functions were used to trigger alerts and notifications based on the analysis of incoming IoT data. This enabled quick responses to issues, preventing potential production disruptions.
Data Lakes and Analytics: Ray Ray Manufacturing stored and analyzed the vast amount of IoT data in AWS data lakes, making it accessible for data scientists and engineers to gain insights into equipment performance and product quality.
Custom Dashboards: They created custom dashboards using AWS services like Amazon QuickSight to visualize manufacturing data in real-time. This allowed operators to monitor the entire production process from a centralized location.